Identify Fit-For-Purpose Data
One of the most common critiques in the regulatory approval process is data that isn’t fit for RWE generation. Too often, relevant variables and endpoints are not captured consistently, or, the data isn’t representative of the target population. Reliable RWE hinges on the selection of fit-for-purpose data and scientifically rigorous analysis, particularly for regulatory-grade studies.
Selecting that fit-for-purpose data, out of the massive amount of healthcare data generated each year, is a tremendous challenge. Fortunately, it’s one that Aetion’s data strategist are passionate about resolving.
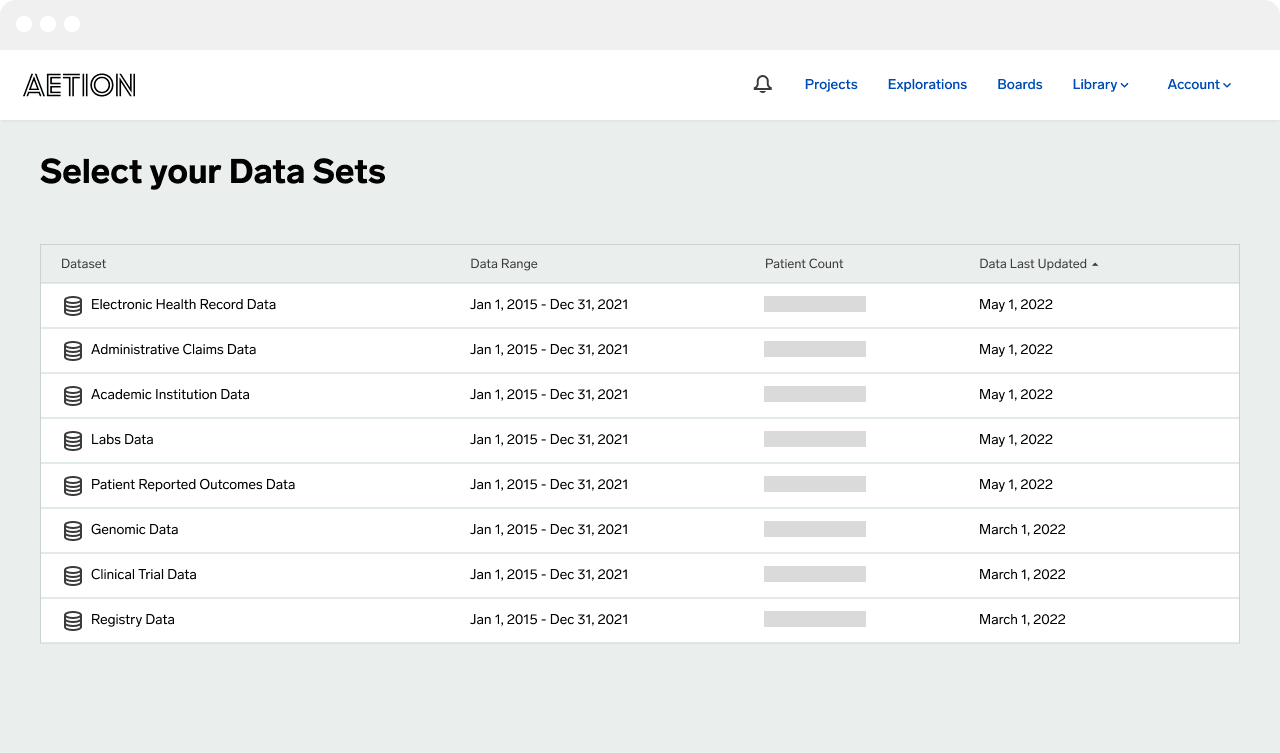
Ensure Suitable Data Investment
Aetion’s data experts understand the landscape and nuances of real-world data, and communicate directly with vendors of real-world data sources, allowing them to make custom recommendations to prioritize data purchasing decisions and increase the likelihood of a client’s success. Aetion’s data services include:
Ensure Suitable Data Investment
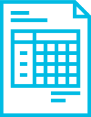
Enterprise data strategy
Assess, prioritize, acquire data for an entire asset portfolio; measure the ROI on data.
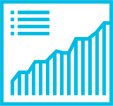
Data landscape evaluation
Understand the landscape of available data sources for a particular therapeutic area or geographic region, and understand how they compare.
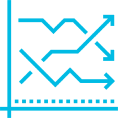
Fit-for-purpose assessment
Using The Structured Process to Identify Fit-for-Purpose Data, (SPIFD) identify which data source(s) are fit-for-purpose for the research question.
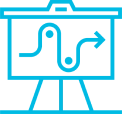
Feasibility assessment
Understand which licensed data source is best to use for a specific study.
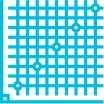
Synthetic data generation
Synthetic data generation. Aetion® Generate and our Privacy & Synthetic Data Services, use artificial intelligence (AI) for synthetic health data generation, ensuring privacy and integrity of the original data source.
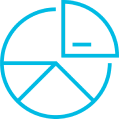
Customizable and reusable measures
Clinical definitions mapping back to the source data can take months to code. Aetion’s data strategists make it easy by providing a library of customizable and reusable algorithms built with the most current clinical definitions.
Establish Data Readiness for Insights
Aetion’s data-fluent scientists can work with any data set, performing rigorous data preparation and integration into Aetion Evidence Platform® (AEP) and unlocking the value of that real-world data to generate critical insights and scalable real-world evidence.
What events do you want to identify?
No criteria have been defined for this measure. Click “Add Filter” to add one.
What events do you want to identify?
No criteria have been defined for this measure. Click “Add Filter” to add one.
What events do you want to identify?
Inpatient
The filter will identify all Inpatient events.